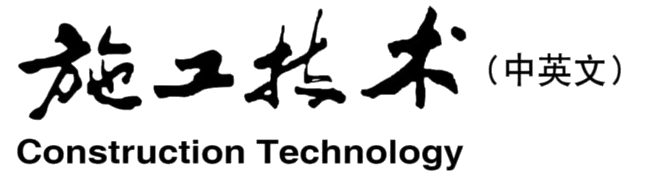

DOI: 10. 7672 / sgjs2024210006
Concrete surface crack detection is an important means of concrete building safety assessmentand risk earlywarning. The traditional manual detection method has a large workload, and it is necessaryto consider factors such as personal safety in complex environments. The crack detection method based onUAV has been applied, but when affected by uncertain factors, UAV cannot collect enough trainingsamples,which limits their detection performance. Therefore, based on UAV and deep learning, a crackdetection method for concrete surface under the condition of few samples is proposed. The mainstreamdeep networks Faster?RCNN and YOLOX are used, and the detection results are fused by WBFalgorithm,which effectively alleviates the detection performance degradation caused by less pixel?levellabel information. Experimental tests were carried out in a few sample crack image library and outdoorsites. The test results show that the performance of the crack detection method based on UAV and deeplearning is effectively improved under the condition of few samples, and the accuracy of crack detection is58. 67%.